What made us say yes: Bringing applied AI to the next level – with Qdrant
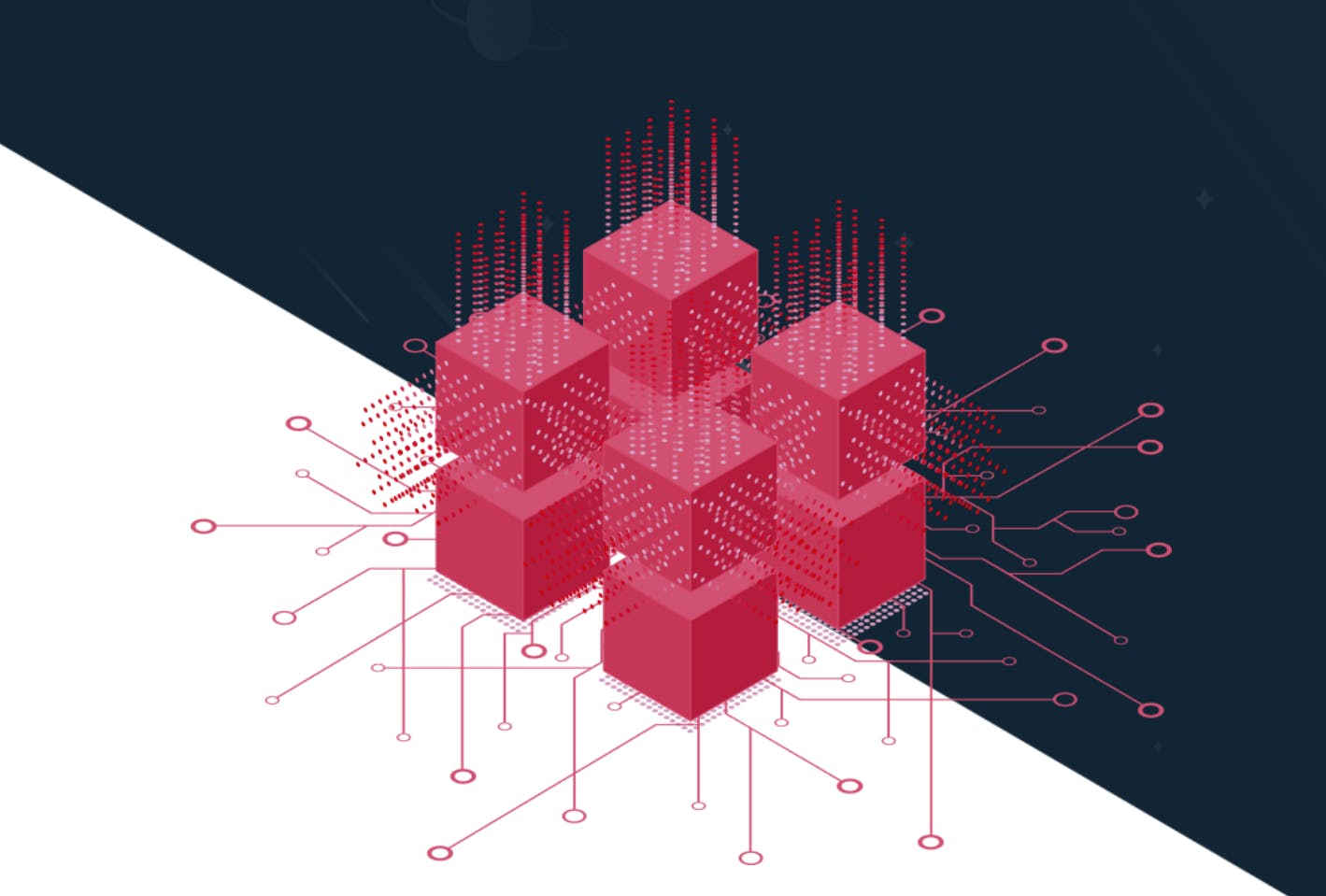
By 2025, 80% of all data is expected to be unstructured, driven by the explosive growth of image, text, video on an ever-increasing number of interfaces (including IoT), with ML models typically transforming unstructured data into feature vectors for data analysis, e.g., product recommendations. Therefore, there is an urgent need for managing high-dimensional vector data in data science and AI applications. Existing vector data management systems and algorithms have two limitations, they
i) have serious performance issues in processing large and dynamic vector data, and
ii) provide limited features that do not meet the needs of versatile applications.
Qdrant addresses these difficulties by being scalable, dynamic, and having an advanced feature set (e.g., attribute filtering, multi-vector queries). Qdrant is a vector similarity engine that deploys as an API service providing search for the nearest high-dimensional vectors. With Qdrant, embeddings or neural network encoders can be turned into full-fledged applications for matching, searching and recommending.
Traditionally, a high manual effort (labeling) is required to train models and only one type of data is considered. Once the models are "trained", updating them requires a lot of effort:
Qdrant addresses these problems methodically with so-called Deep Metric Learning (DML). In DML, objects are assigned vectors based on rules or complex functions, whereby semantic proximity (and thus the distance between different objects) is mapped. With Qdrant's technology, no complex labeling is required and models can be adapted without much effort. In addition, datasets of different types can be combined, which is necessary for unstructured databases. This makes high-performance DML applications applicable to a broader target group with less resource input.
Importantly, Qdrant is the only solution with advanced filtering capabilities and a distributed, cloud-native deployment that scales horizontally. Therefore, no matter how much data you need to serve Qdrant can always be used with just the right amount of computational resources.
This space is evolving at a high rate making collaboration and transparency necessary, this is why Qdrant is a COSS (Commercial Open Source Software) enabling others to try/test/build with Qdrant. There are many applications still to be built and we look forward to seeing what the community around Qdrant builds using the evolving infrastructure.